Temperature and day-length are considered to be the 2 important factors that can significantly affect insect diapause, which is a typical proportional dataset. In the previous studies, the method of arcsine square root transformation is widely used to analyze the effect of temperature or day-length or their joint effects on diapause in insects. However, this method has many limitations, for example, the proportional data should be normally distributed. The logistic regression in generalized additive models is a promising method for analyzing the effects of temperature and day-length on diapause. Compared to the arcsine square root transformation method, this method does not require normal distribution of proportional diapause data. The logistic regression also provides better goodness-of-fit by using the non-parametric fitting technique. In this report, we used the diapause data of Pieris melete (Xiao et al. 2012) to compare the fitted results of the logistic regression in generalized additive models with arcsine square root transformation. We found that the logistic regression in generalized additive models is better than linear regression of arcsine square root transformed data in following ways: (1) reasonable predictions about diapause ranging from 0 to 1 can be made without transforming the proportional data; (2) non-linear effects of temperature and day-length on diapause can be determined; (3) the goodness-of-fit can be substantially improved.
How to translate text using browser tools
1 September 2013
Logistic Regression is a better Method of Analysis Than Linear Regression of Arcsine Square Root Transformed Proportional Diapause Data of Pieris melete (Lepidoptera: Pieridae)
P. J. Shi,
H. S. Sand Hu,
H. J. Xiao
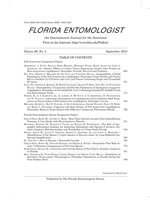
Florida Entomologist
Vol. 96 • No. 3
September 2013
Vol. 96 • No. 3
September 2013
additive model
binomial response variables
conjunto de datos proporcionales
efectos no lineales
goodness-of-fit
grado de ajuste
modelo aditivo